Posted by Aseem Agarwala, Research Scientist, Clips Content Team LeadTo me, photography is the simultaneous recognition, in a fraction of a second, of the significance of an event as well as of a precise organization of forms which give that event its proper expression.—
Henri Cartier-BressonThe last few years have witnessed a Cambrian-like explosion in AI, with deep learning methods enabling computer vision algorithms to recognize many of the elements of a good photograph: people, smiles, pets, sunsets, famous landmarks and more. But, despite these recent advancements, automatic photography remains a very challenging problem. Can a camera capture a great moment automatically?
Recently, we released
Google Clips, a new, hands-free camera that automatically captures interesting moments in your life. We designed Google Clips around three important principles:
- We wanted all computations to be performed on-device. In addition to extending battery life and reducing latency, on-device processing means that none of your clips leave the device unless you decide to save or share them, which is a key privacy control.
- We wanted the device to capture short videos, rather than single photographs. Moments with motion can be more poignant and true-to-memory, and it is often easier to shoot a video around a compelling moment than it is to capture a perfect, single instant in time.
- We wanted to focus on capturing candid moments of people and pets, rather than the more abstract and subjective problem of capturing artistic images. That is, we did not attempt to teach Clips to think about composition, color balance, light, etc.; instead, Clips focuses on selecting ranges of time containing people and animals doing interesting activities.
Learning to Recognize Great MomentsHow could we train an algorithm to recognize interesting moments? As with most machine learning problems, we started with a dataset. We created a dataset of thousands of videos in diverse scenarios where we imagined Clips being used. We also made sure our dataset represented a wide range of ethnicities, genders, and ages.
We then hired expert photographers and video editors to pore over this footage to select the best short video segments. These early curations gave us examples for our algorithms to emulate. However, it is challenging to train an algorithm solely from the subjective selection of the curators — one needs a smooth gradient of labels to teach an algorithm to recognize the quality of content, ranging from "perfect" to "terrible."
To address this problem, we took a second data-collection approach, with the goal of creating a continuous quality score across the length of a video. We split each video into short segments (similar to the content Clips captures), randomly selected pairs of segments, and asked human raters to select the one they prefer.
We took this pairwise comparison approach, instead of having raters score videos directly, because it is much easier to choose the better of a pair than it is to specify a number. We found that raters were very consistent in pairwise comparisons, and less so when scoring directly. Given enough pairwise comparisons for any given video, we were able to compute a continuous quality score over the entire length. In this process, we collected over 50,000,000 pairwise comparisons on clips sampled from over 1,000 videos. That’s a lot of human effort!
Training a Clips Quality ModelGiven this quality score training data, our next step was to train a neural network model to estimate the quality of any photograph captured by the device. We started with the basic assumption that knowing what’s
in the photograph (e.g., people, dogs, trees, etc.) will help determine “interestingness”. If this assumption is correct, we could learn a function that uses the recognized content of the photograph to predict its quality score derived above from human comparisons.
To identify content labels in our training data, we leveraged the same Google machine learning technology that powers Google image search and Google Photos, which can
recognize over 27,000 different labels describing objects, concepts, and actions. We certainly didn’t need all these labels, nor could we compute them all on device, so our expert photographers selected the few hundred labels they felt were most relevant to predicting the “interestingness” of a photograph. We also added the labels most highly correlated with the rater-derived quality scores.
Once we had this subset of labels, we then needed to design a compact, efficient model that could predict them for any given image, on-device, within strict power and thermal limits. This presented a challenge, as the deep learning techniques behind computer vision typically require strong desktop GPUs, and algorithms adapted to run on mobile devices lag far behind state-of-the-art techniques on desktop or cloud. To train this on-device model, we first took a large set of photographs and again used Google’s powerful, server-based recognition models to predict label confidence for each of the “interesting” labels described above. We then trained a
MobileNet Image Content Model (ICM) to mimic the predictions of the server-based model. This compact model is capable of recognizing the most interesting elements of photographs, while ignoring non-relevant content.
The final step was to predict a single quality score for an input photograph from its content predicted by the ICM, using the 50M pairwise comparisons as training data. This score is computed with a
piecewise linear regression model that combines the output of the ICM into a frame quality score. This frame quality score is averaged across the video segment to form a moment score. Given a pairwise comparison, our model should compute a moment score that is higher for the video segment preferred by humans. The model is trained so that its predictions match the human pairwise comparisons as well as possible.
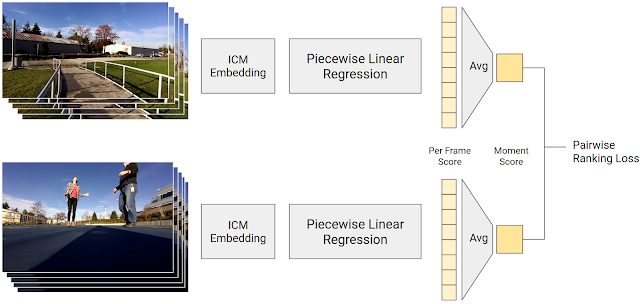 |
Diagram of the training process for generating frame quality scores. Piecewise linear regression maps from an ICM embedding to a score which, when averaged across a video segment, yields a moment score. The moment score of the preferred segment should be higher. |
This process allowed us to train a model that combines the power of Google image recognition technology with the wisdom of human raters–represented by 50 million opinions on what makes interesting content!
While this data-driven score does a great job of identifying interesting (and non-interesting) moments, we also added some bonuses to our overall quality score for phenomena that we know we want Clips to capture, including faces (especially recurring and thus “familiar” ones), smiles, and pets. In our
most recent release, we added bonuses for certain activities that customers particularly want to capture, such as hugs, kisses, jumping, and dancing. Recognizing these activities required extensions to the ICM model.
Shot ControlGiven this powerful model for predicting the “interestingness” of a scene, the Clips camera can decide which moments to capture in real-time. Its shot control algorithms follow three main principles:
- Respect Power & Thermals: We want the Clips battery to last roughly three hours, and we don’t want the device to overheat — the device can’t run at full throttle all the time. Clips spends much of its time in a low-power mode that captures one frame per second. If the quality of that frame exceeds a threshold set by how much Clips has recently shot, it moves into a high-power mode, capturing at 15 fps. Clips then saves a clip at the first quality peak encountered.
- Avoid Redundancy: We don’t want Clips to capture all of its moments at once, and ignore the rest of a session. Our algorithms therefore cluster moments into visually similar groups, and limit the number of clips in each cluster.
- The Benefit of Hindsight: It’s much easier to determine which clips are the best when you can examine the totality of clips captured. Clips therefore captures more moments than it intends to show to the user. When clips are ready to be transferred to the phone, the Clips device takes a second look at what it has shot, and only transfers the best and least redundant content.
Machine Learning FairnessIn addition to making sure our video dataset represented a diverse population, we also constructed several other tests to assess the fairness of our algorithms. We created controlled datasets by sampling subjects from different genders and skin tones in a balanced manner, while keeping variables like content type, duration, and environmental conditions constant. We then used this dataset to test that our algorithms had similar performance when applied to different groups. To help detect any regressions in fairness that might occur as we improved our moment quality models, we added fairness tests to our automated system. Any change to our software was run across this battery of tests, and was required to pass. It is important to note that this methodology can’t guarantee fairness, as we can’t test for every possible scenario and outcome. However, we believe that these steps are an important part of our long-term work to achieve
fairness in ML algorithms.
ConclusionMost machine learning algorithms are designed to estimate objective qualities – a photo contains a cat, or it doesn’t. In our case, we aim to capture a more elusive and subjective quality – whether a personal photograph is interesting, or not. We therefore combine the objective, semantic content of photographs with subjective human preferences to build the AI behind Google Clips. Also, Clips is designed to work alongside a person, rather than autonomously; to get good results, a person still needs to be conscious of framing, and make sure the camera is pointed at interesting content. We’re happy with how well Google Clips performs, and are excited to continue to improve our algorithms to capture that “perfect” moment!
AcknowledgementsThe algorithms described here were conceived and implemented by a large group of Google engineers, research scientists, and others. Figures were made by Lior Shapira. Thanks to Lior and Juston Payne for video content.