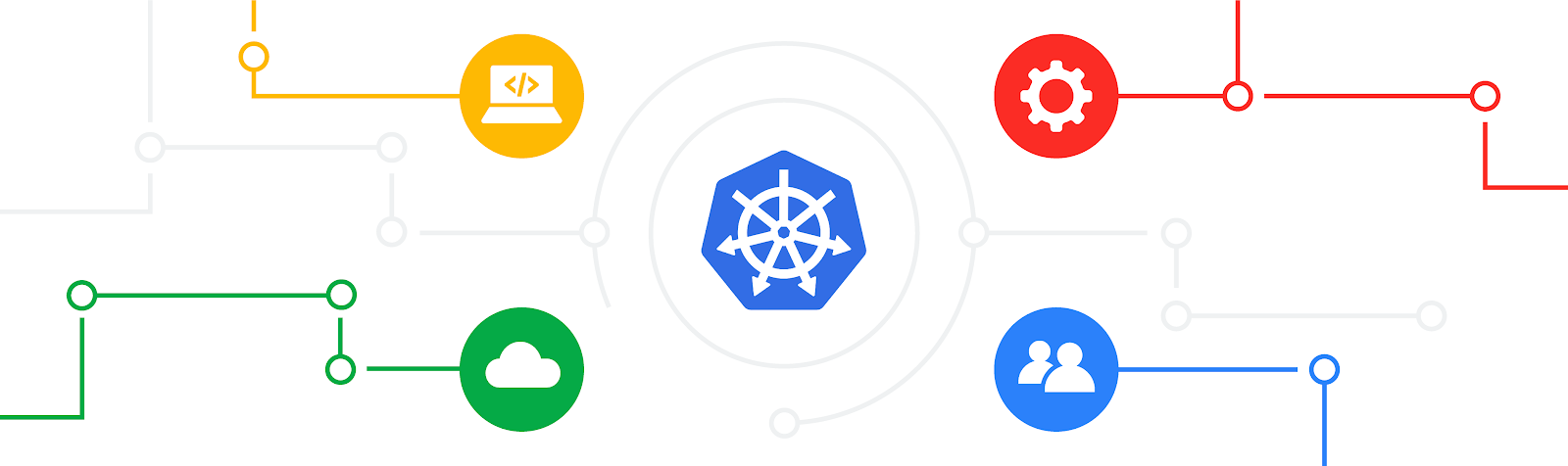
I am thrilled to introduce Common Expression Language, a simple expression language that's great for writing small snippets of logic which can be run anywhere with the same semantics in nanoseconds to microseconds evaluation speed. The launch of cel.dev marks a major milestone in the growth and stability of the language.
It powers several well known products such as Kubernetes where it's used to protect against costly production misconfigurations:
- object.spec.replicas <= 5
Cloud IAM uses CEL to enable fine-grained authorization:
- request.path.startsWith("/finance")
Versatility
CEL is both open source and openly governed, making it well adopted both inside and outside Google. CEL is used by a number of large tech companies, either internally or as part of their public product offering. As a reflection of this open governance, cel.dev has been launched to share more about the language and the community around it.
So, what makes CEL a good choice for these applications? Why is CEL unique or different from Amazon's Cedar Policy or Open Policy Agent's Rego? These are great questions, and common ones (no pun intended):
- Highly optimized evaluation O(ns) - O(μs)
- Portable with stacks supported in C++, Java, and Go
- Thousands of conformance tests ensure consistent behavior across stacks
- Supports extension and subsetting
Subsetting is crucial for preserving predictable compute / memory impacts, and it only exists in CEL. As any latency-critical service maintainer will tell you, it's vital to have a clear understanding of compute and memory implications of any new feature. Imagine you've chosen an expression language, validated its functionality meets your security, serving, and scaling goals, but after launching an update to the library introduces new functionality which can't be disabled and leaves your product vulnerable to attack. Your alternatives are to fork the library and accept the maintenance costs, introduce custom validation logic which is likely to be insufficient to prevent abuse, or to redesign your service. CEL supported subsetting allows you to ensure that what was true at the initial product launch will remain true until you decide when to expose more of its functionality to customers.
Cedar Policy language was developed by Amazon. It is open source, implemented in Rust, and offers formal verification. Formal verification allows Cedar to validate policy correctness at authoring time. CEL is not just a policy language, but a broader expression language. It is used within larger policy projects in a way that allows users to augment their existing systems rather than adopt an entirely new one.
Formal verification often has challenges scaling to large amounts of data and is based on the belief that a formal proof of behavior is sufficient evidence of compliance. However, regulations and policies in natural language are often ambiguous. This means that logical translations of these policies are inherently ambiguous as well. CEL's approach to this challenge of compliance and reasoning about potentially ambiguous behaviors is to support evaluation over partial data at a very high speed and with minimal resources.
CEL is fast enough to be used in networking policies and expressive enough to be used in detailed application policies. Having a greater coverage of use cases improves your ability to reason about behavior holistically. But, what if you don't have all the data yet to know exactly how the policy will behave? CEL supports partial evaluation using four-valued logic which makes it possible to determine cases which are definitely allowed, denied, or where policy behavior is conditional on additional inputs. This allows for what-if analysis against historical data as well as against speculative data from new use cases or proposed policy changes.
Open Policy Agent's Rego is also open source, implemented in Golang and based on Datalog, which makes it possible to offer similar proofs as Cedar. However, the language is much more powerful than Cedar, and more powerful than CEL. This expressiveness means that OPA Rego is fantastic for non-latency critical, single-tenant solutions, but difficult to safely embed in existing offerings.
Four-valued Logic
CEL uses commutative logical operators that can render a true, false, error, or unknown status. This is a scalable alternative to formal verification and the expressiveness of Datalog. Four-valued logic allows CEL to evaluate over a partial set of inputs to deliver either a definitive result or communicate that more information is necessary to complete the evaluation.
What is four-valued logic?
True, false, and error outcomes are considered definitive: no additional information will change the outcome of the expression. Consider the following case:
- 1/0 != 0 && false
In traditional programming languages, this expression would be an error; however, in CEL the outcome is false.
Now consider the following case where an input variable, called unknown_var is marked as unknown:
- unknown_var && true
The outcome of this expression is UNKNOWN{unknown_var} indicating that once the variable is known, the evaluation can be safely completed. An unknown indicates what was missing, and alerts the user to fix the outcome with more information. This technique both borrows from and extends SQL three-valued predicate logic which uses TRUE, FALSE, and NULL with commutative logical operators. From a CEL perspective, the error state is akin to SQL NULL that arises when there is an absence of information.
CEL compatibility with SQL
CEL leverages SQL semantics to ensure that it can be seamlessly translated to SQL. SQL optimizers perform significantly better over large data sets, making it possible to evaluate over data at rest. Imagine trying to scale a single expression evaluation over tens of millions of records. Even if the expression evaluates within a single microsecond, the evaluation would still take tens of seconds. The more complex the expression, the greater the latency. SQL excels at this use case, so translation from CEL to SQL was an important factor in the design in order to unlock the possibility of performant policy checks both online with CEL and offline with SQL.
Thank you CEL Community!
We’re proud to announce cel.dev as a major milestone in the maturity and adoption of the language, and we look forward to working with you to make CEL the best building block for writing latency-critical, portable logic. Feel free to contact us at [email protected]
By Tristan Swadell – Senior Staff Software Engineer